From Risk To Retention Transforming Insurance With Predictive Churn Analytics prior to, the primary responsibilities of insurance companies were evaluating risks, policy underwriting, and claims handling. But the environment has changed significantly. Customer retention is a key challenges that agencies confront nowadays. The stakes are bigger than ever because customers have many options and can swiftly switch providers.
Realizing that it may be far more expensive to acquire a new customer than to keep an existing one is what has caused this shift in emphasis. Additionally, loyal consumers frequently have higher lifetime values and can act as ambassadors for the company, fostering organic growth through favorable word-of-mouth. In this situation, it has become critically critical for insurance businesses to learn the reasons behind potential customer attrition and take proactive measures to avoid it.
Understanding Predictive Churn Analytics
Insurance firms can now zero in on proactive customer retention strategies as opposed to traditional risk assessment, thanks to predictive churn analytics, which has totally transformed the industry. Using big data, machine learning, and powerful methods of statistical analysis, this novel the method predicts which policyholders are most likely to stop their coverage. Insurers might improve retention rates and ensure a steady customer base by taking specific steps to identify these at-risk consumers early on.
The Importance of Customer Retention
It is tough to oversell the significance of client retention in the insurance sector. Sustaining current policyholders is typically less expensive than acquiring new ones, and policyholders with a longer duration usually produce higher profits. Furthermore, retention has become essential to preserving market share and profitability in an increasingly competitive sector where consumers can quickly compare costs and switch suppliers online.
Insurers who used complex retention tactics, like as predictive churn analytics, had an average 23% improve in customer lifetime value over those who did not, according to RetentionMetrics’ 2024 Insurance Customer Loyalty Report.
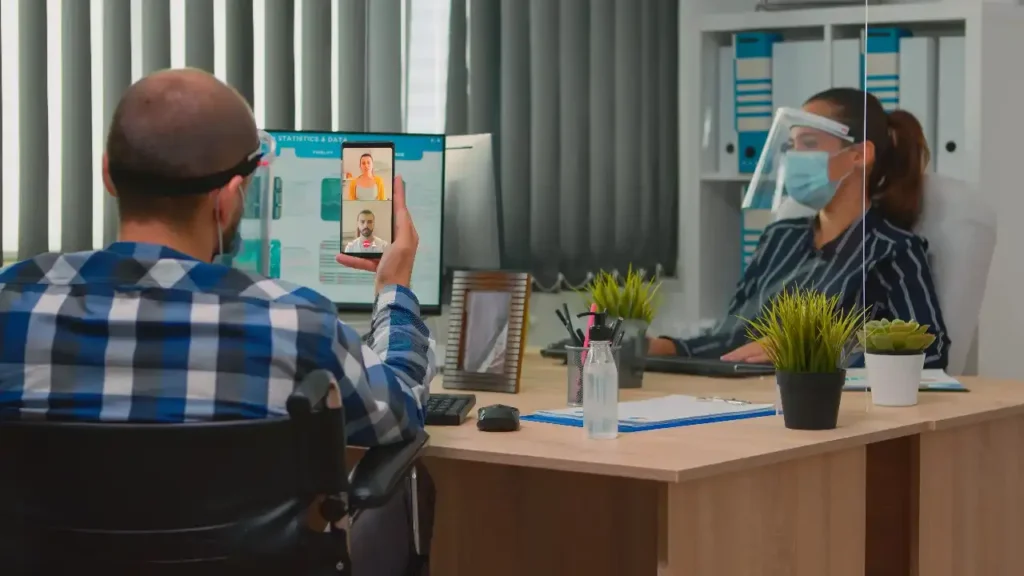
How Predictive Churn Analytics Works
Large volumes of data from numerous sources, such as policy information, customer demographics, interaction data, and outside variables, are analyzed in order to do predictive churn analytics. Through the use for complicated algorithms, insurers are able to analyze this data and find trend and risk factors related to client attrition. These insights help businesses to divide their policyholder base according on churn risk and develop comprehensive customer profiles. Insurance providers can create customized retention strategies for different types of customers thanks to this segmentation.
Large volumes of data from numerous sources, such as policy information, customer demographics, interaction data, and additional variables, are processed in order to do predictive churn analytics. Through the use of complex algorithms, insurers have the capacity to analyze this data and find trends and risk factors related to client attrition. These insights enable firms to develop complete client profiles and divide their
The Power of Data in Churn Prediction
The caliber and quality of data that is obtainable significantly affects how effective predicted churn analytics are. To create precise prediction models, insurers use a variety of data points. This includes past policy data, prior claims background, interactions with consumers, and even other factors like competitive activity and economic indicators. The churn estimates get more precise the more complete and accurate the data are.
The Role of Synthetic Data in Churn Analytics
A rapidly developing area of study called data science has led to a number of predictive analytics programs that use data to help achieve better business decisions. To assist policyholders acquire the most protection at the best price and better manage their risk, the insurance sector, for instance, uses data from agent interactions, customer interactions, smart homes, massive data sets, loss information, and telematics.
Predictive churn analytics also depends heavily on machine learning techniques. Complex patterns and linkages in the data that human analysts might not notice can be found by these algorithms. These computer models get better at forecasting buyer habits as they process more data over time. This skill is further improved by artificial intelligence, which makes real-time analysis and automation
Key Benefits of Predictive Churn Analytics
Predictive churn analytics may give insurance companies with a competitive edge, better consumer experiences, targeted interventions, effective resource allocation, and product development. Insurance firms can improve customer satisfaction by offering greater level of customization and communication by getting to know each individual’s needs and preferences. Businesses might focus their retention efforts on high-risk clients, providing rewards or revising their products to make them stay.
Personalizing Customer Experiences
The capacity to adapt customer experiences is among predictive churn analytics’ most important capabilities. Insurance businesses may tailor their offers, communications, and services by knowing the specific specifications, preferences, and risk factors of each policyholder. In addition to boost customer happiness, this degree of modification builds up the bond between the policyholder and the insurer, reducing chance that the policyholder may move providers.
Proactive Risk Management
Insurers can also manage risk more proactively thanks to predictive churn analytics. Firms can keep customers from choosing to leave by recognizing possible churners early on. This might mean confronting complaints directly, proposing changes to the policy, or giving extra value-added services. These measures can greatly lower attrition rates and increase total client retention.
Implementation Challenges and Requirements
- Lack of technical expertise hinders effective data utilization.
- Employee resistance to change hinders adoption of predictive analytics.
- Investment in technology and expertise is required for advanced data analytics platforms, data scientists, and system integration.
- Potential return on investment is substantial, improving retention rates and customer lifetime value.
- Increased volume, variety, and velocity of data in hyperconnected world transforms business operations.
Overcoming Data Privacy Concerns
In the face increasing customer data collection and analysis by insurers, privacy concerns must be addressed. Firms need to make sure they follow data protection laws and have open policies about how they use customer data. For these programs to be productive in the long run, it is imperative that customers have trust within the way their data is used for predictive analytics.
Best Practices for Maximizing Benefits
Insurance organizations should adhere to best practices, which include verifying data quality, merging multiple data sources, updating models often, acting on insights, tracking and assessing results, and upholding ethical standards, in order to optimize the benefits of predictive churn analytics. These procedures assist insurers in keeping precise forecasts, obtaining thorough insights into policyholder behavior, and adjusting to shifting market circumstances.
Integrating Churn Analytics with Other Business Processes
Predictive churn analytics must be related to other business processes in order to be actually effective. This covers manufacturing of products, promotional strategies, and support for clients. Through combining of churn analytics insights with these operations, insurers can build a comprehensive client retention strategy that is embraced by the entire organization.
In a Nutshell,
Despite all the celebration, indicated churn analytics today signifies a big change in the way insurance firms handle client relationships. Insurance organizations can change from reactive risk management to proactive customer retention by utilizing data and advanced analytics. This shift improves consumer satisfaction and loyalty in addition to improving the bottom line. Predictive churn analytics is certain to grow more and more important in guiding the direction of the insurance sector as technology develops.
FAQS
What are the Best Practices for Maximizing Benefits?
Insurance organizations should use best practices like data quality verification, model updates, insights assessment, and ethical standards to optimize predictive churn analytics for accurate forecasts and market adjustments.
What are the Implementation Challenges and Requirements?
Technical expertise and employee resistance hinder data utilization and predictive analytics adoption. Investment in technology and expertise improves retention rates and customer lifetime value.